Streamlining Investment Operations with Intelligent Automation
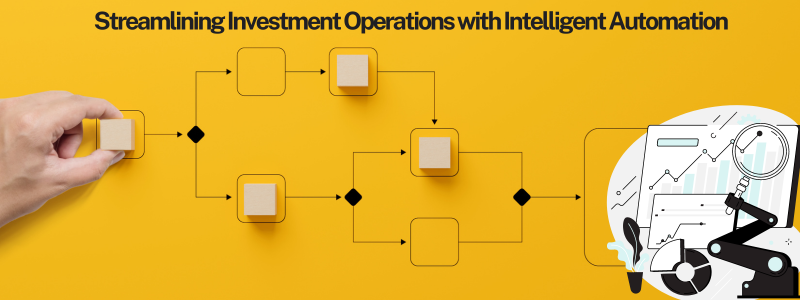
The world of investment operations has been stuck in a time warp for years - relying on manual processes across siloed teams, driven by spreadsheets and endless email chains. But in our post-COVID reality, it's clear this status quo just doesn't cut it anymore.
With markets swinging wildly day-to-day and investors demanding hyper-personalization, the strains on operations teams have reached a boiling point. Firms now realize tools and platforms need some serious upgrading and integration to keep pace with the complexity of the interconnected business.
The industry is now at the cusp of a new era of intelligent automation - one that promises improved efficiency, consistency, and scalability. The potential? Nothing short of a revolution in how investment teams could collaborate to drive better portfolio performance.
This transformation won't happen overnight. But forward-thinking firms are already exploring how intelligent automation can help consolidate fragmented systems and streamline operations while boosting security, control, and governance. They know standing still amidst disruption isn't an option if they want a competitive advantage.
In fact, over 67% of financial services companies are currently adopting automation to some degree, with 21% planning to automate a quarter of banking roles by 2025. With such tremendous growth and potential, intelligent automation is undoubtedly reshaping the future of investment operations.
Let's explore key considerations around implementing intelligent automation for investment operations in 2024 - from the tangible benefits to responsible adoption to choosing the right solutions.
Benefits of Automating Investment Operations
The imperative for efficiency and integration in investment operations necessitates a hard look at intelligent automation. Automating hitherto manual processes in investment operations confers multifarious benefits that categorically make a compelling case for its adoption.
- Consistency in Processes and Data: First up, automation brings some much-needed consistency in processes and data. No more ambiguity from teams winging it on their own workflows. Preset rules standardize everything - from compiling data to calculations to reporting.
- Enhanced Scalability to Handle a Growing Volume of Transactions: Scalability is another major win. With trade volumes and asset classes booming, flexible software robots can effortlessly scale up or down to handle fluctuating workloads. This scalability enhances operational resilience even in periods of extreme market volatility.
- Increased Efficiency through Standardized Workflows: Standardizing workflows also directly boosts efficiency by cutting out redundancies and lags. Tasks get knocked out faster as automation software optimizes sequences and unblocks bottlenecks. No fatigue either - automated systems run 24/7 at full throttle. McKinsey estimates a 60-70% reduction in process turnaround time through automation.
- Cost Savings from Reduced Manual Labor and Human Errors: Cost optimization is another compelling benefit, given automation obviates manual labor and minimizes errors. Automating manual tasks slashes operational costs by 30-60%, per McKinsey. Apart from sizable labor cost savings, automation mitigates losses from penalties, fines, and settlements stemming from human errors. It also boosts STP rates, translating to higher rebates.
- Real-Time Data for Informed Decision Making: Accessing real-time data is invaluable too. Automated systems enable instant data collection and analysis, so decision-makers can swiftly respond to market shifts. That agility is especially vital in fast-changing APAC.
AI and Robotics for Automation
Bringing AI and robotics into investment operations isn't just about the future - it's already reshaping how firms work today. These technologies are revolutionizing repetitive yet critical tasks, setting a new bar for efficiency and accuracy.
- Software Robots for Repetitive Data Tasks: Software robots can take over mundane data jobs like trade capture, data entry, aggregating account info, and rebalancing portfolios. Their tireless consistency handles high volumes with ease, boosting speed and accuracy.
- Machine Learning for Pattern Recognition: Machine learning algorithms can detect patterns in transaction data to spot anomalies, model optimal trading strategies, or forecast moves in volatile markets. As firms generate more data, machine learning will become indispensable for pattern recognition and predictive analytics.
- Natural Language Processing for Enhanced Customer Interaction: Conversational interfaces powered by natural language processing allow customers and employees to query portfolios, submit service requests, or access reporting through voice commands or chatbots. These interfaces simplify interactions and provide quick, personalized responses.
- Streamlining Trade Confirmation and Settlement: Mundane back-office processes like trade confirmation and settlement, corporate actions processing, and reconciliations can also benefit from straight-through processing through automated workflows. Exceptions are seamlessly routed to appropriate teams.
- Data Management: We just can't overlook AI and robotics for data management. With the ability to organize, analyze, and secure vast data, these technologies form the backbone of data-driven investment strategies, helping firms tap their full informational potential.
Integrating these automated solutions cements intelligent automation as a game-changer for investment operations. Firms that embrace AI and robotics will gain a distinct edge over the competition.
Regulatory Considerations with AI
As investment operations race to tap automation and AI, it's vital to keep ethics and regulations in front of mind.
The financial sector navigates complex oversight, and new tech brings fresh challenges around transparency, bias, and security. Regulators globally and in the APAC region are still playing catch-up on AI governance. But firms can take key steps to ensure responsible AI:
- Ensuring Transparency and Explainability: A key priority is to address AI's "black box" problem - its opacity around how algorithms arrive at decisions. Regulators increasingly expect explainability around AI's workings to ensure accountability. Firms should invest in techniques like LIME that produce explanations for AI model outputs.
- Testing for Unintended Bias: Bias in data or algorithms can propagate unfair outcomes. Rigorously testing and monitoring AI systems for demographic biases is essential to avoid discriminatory investment decisions or barriers to financial inclusion.
- Incorporating Human Oversight: While automation delivers efficiency, retaining human oversight over AI is critical, especially for high-risk processes. Human-in-the-loop systems with manual checkpoints and override abilities can counter automation bias risks.
- Prioritizing Data Security: Vast data collection and analysis heighten vulnerabilities like cyber-attacks or privacy breaches. Following robust data governance practices in AI development and deployment is mandatory, particularly with sensitive client data.
- Adopting Ethical AI Frameworks: Voluntary governance frameworks like Singapore's FEAT principles guide firms to ensure AI aligns with ethical values around transparency, fairness, and accountability
The goal is to tap AI's power while upholding ethics and sound oversight. Prioritizing responsibility now saves regulatory headaches down the road.
Disruptors and New Technologies
The investment operations landscape is undergoing a metamorphosis, with new disruptive technologies and business models challenging the status quo. As we look ahead to InvestOps Asia 2024, some key disruptors and technologies set to transform investment operations include:
- Cloud-Based Wealth Management Platforms: Cloud-based wealth management platforms are displacing legacy systems with low-cost, flexible solutions for portfolio management, trading, reporting, and more. Leading platforms like BlackRock's Aladdin are enabling smaller firms to access enterprise-grade capabilities without massive IT investments.
- Automated Advisory Services: "Robo-advisors" using algorithms and AI for automated portfolio management and advisory are making wealth management accessible to wider investment segments. China's Futu has over 20 million clients leveraging its digitized, low-cost advisory. As robo-advisory matures, hybrid human-AI models will likely dominate.
- Blockchain for Transactions and Digital Assets: Blockchain is revolutionizing asset and data exchange in capital markets. Instead of days, settlement finality in minutes reduces risk. Australia Stock Exchange uses blockchain to supercharge transactions. Cryptocurrency adoption also keeps climbing across APAC. In 2022, Vietnam had the highest share of cryptocurrency adopters in the APAC region at 41%, followed by the Philippines at 32% and India at 30%. Comparatively, more developed economies like Singapore and Japan had lower but still significant adoption rates of 28% and 27% respectively.
- Big Data and AI for Decision-Making: Sophisticated AI algorithms leveraging alternative datasets from IoT sensors, satellites, and more are enhancing predictive analytics and investment decisions. Chinese firm Ping An Good Doctor trains AI on 310 million+ medical records to inform life and health insurance products.
While disruptive, these innovations also create opportunities to transform operations, unlock efficiencies and better serve customers. Savvy leaders see them not as threats, but as tools to cement competitive advantage.
Build vs Buy Considerations for AI in Investment Operations
So you're an investment firm keen to jump on board the AI train. The big question - should you build your own customized AI solutions in-house or buy pre-made ones from vendors? Solid arguments on both sides here.
Building in-house lets you highly customize systems to your firm's specific needs. However, it often demands extra upfront investment in data infrastructure and AI talent. Development can get complex too if you lack experience rolling out AI.
Off-the-shelf vendor solutions mean faster deployment and lower costs out the gate. However, they offer less flexibility to tailor platforms to your unique processes or data.
A third option? Take a phased approach - first, buy a vendor package to score some quick wins. Then later customize it in-house once your team is trained up. This way you balance speedy deployment with customization down the road.
Finally, consider an AI consultancy partnership. Collaborate first to shape an AI strategy, then co-build solutions before full rollout. This bakes in customization for long-term needs together with vendor rollout experience.
As firms move towards intelligent automation, evaluating these build vs buy tradeoffs will shape the most viable AI adoption strategy - one aligned with available resources, capabilities, and strategic priorities.
Conclusion
As we stand at the dawn of a new age in investment ops, integrating intelligent automation, AI and robotics is no longer some far-off vision — it's already here. The talks and insights from InvestOps Asia will surely light the path ahead for COOs, heads of ops, and tech leaders across APAC.
Of course, the road towards full automation has its bumps. But for firms that harness these tools successfully, the payoff is huge. They'll not only ace the current climate but also lay the foundations for continued innovation down the line.